
Upscaling Global Hourly GPP with Temporal Fusion Transformer (TFT)
Accepted Oral Paper at CVPR 2023 MultiEarth Workshop
A MIDS Capstone Project in Collaboration with
The Quantitative Ecosystem Dynamics Lab at UC Berkeley
Carbon Chaser
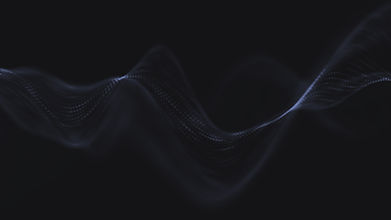
Challenges around
Global Hourly GPP Upscale
Gross Primary Productivity (GPP) quantifies plant's carbon uptake and photosynthesis activities, which are crucial to regulating the Earth's climate and reducing carbon emissions. However, accurately measuring hourly GPP at a global scale has been challenging..
Limited Flux Towers
GPP is measured by flux towers, but there is only 263 sites around the world measuring GPP and most are located in N. America and Europe.
High Data Variance
GPP measurements can varies greatly during a day, or among different sites. Such characteristic make it difficult for a single model to capture such intricacies.
Lack of High-Granularity Data
Few data around this problem are recorded in hourly or finer resolution. Most are recorded in lower resolution (i.e. daily, weekly, or monthly).
Limited Application with Modern ML Models
Current state-of-the-art model are either process-based model based the real-world physics theories, or lack awareness about long-term temporal dependency among data
Key Research Accomplishment
With the goal of improving model performance of global hourly GPP upscale through Temporal Fusion Transformer, our team was able accomplish the following:
Model Outperformed
Past Studies
Our best model out preformed past studies by 2% NSE and 10% RMSE.
Unconventional Application of Temporal Fusion Transformer
Typical time-series forecast required dataset with available past target values. This study successfully applied TFT to timer-series forecast without past target values.
New Analytics on Temporal Dynamics of the Predictors
Derived from TFT's feature importance and attention weights, the study provide new insights into the temporal dynamics of feature significance for each prediction.


Apply
Temporal Fusion Transformer to Upscale
Hourly Global GPP
Temporal Fusion Transformer (TFT) is an attention-based time-series forecasting model is proposed by Google Research around 2018. It possess novel features, including:
-
Learns short- and long- term patterns through LSTM and self-attention mechanisms.
-
Support heterogeneous time series.
-
Provides interpretable insights into temporal dynamics in the data.
We aim to overcome the limitations of current GPP data products and create higher-performing and interpretable models and contribute to scientific research in this area and provide new insights through the capabilities of Temporal Fusion Transformer (TFT).
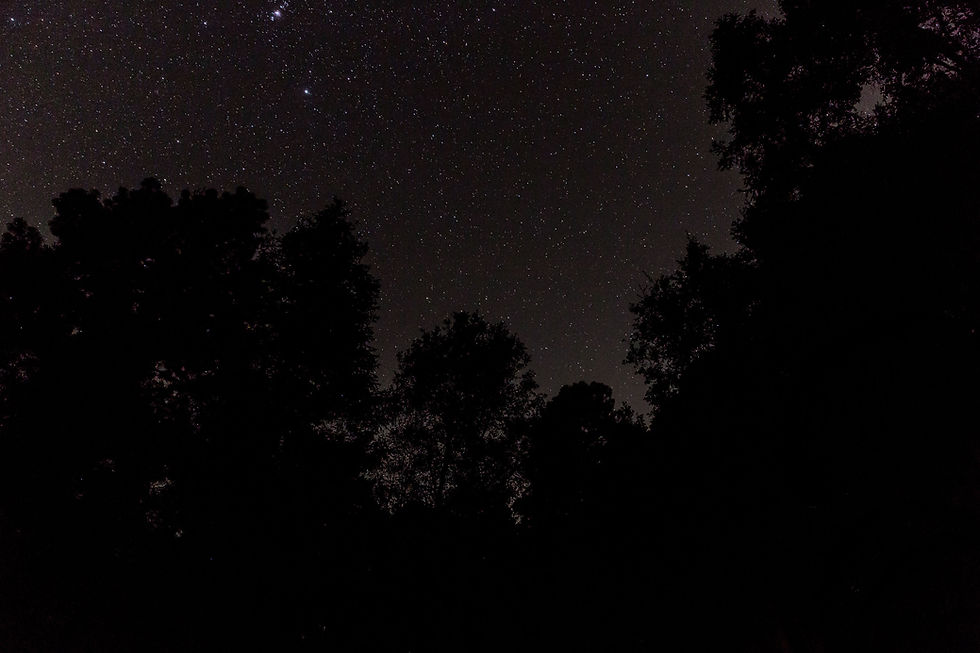
About Our Data
The target features of this research is the GPP measuremet from 129 sites from FLUXNET, a network of flux tower sites that each collect various ecological measurements and pull together public datasets for the global research community. These flux tower sites span acriss the globe but are primarily concentrated in the United States and Europe. Though valuable predictor features are provided by the FLUXNET datasets, only features that are globally available can be used for training upscaling models. Therefore, a wide set of globally available meteorological and remote sensing datasets, each with varying temporal and spatial resolutions were explored for use in modeling
5
Years (2010-2015)
192
Flux Towers
4.6M
Total Records
12
IGBP Types
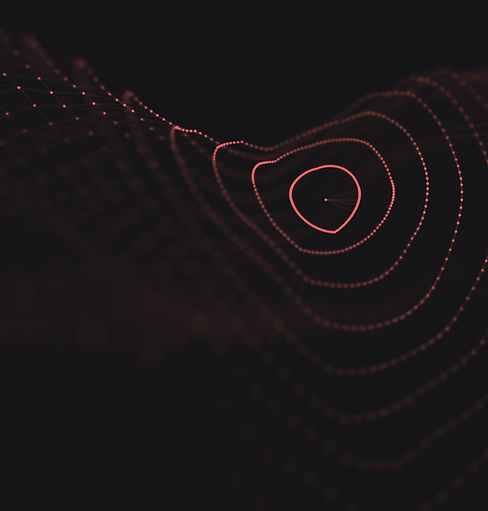
Model Experiments with Temporal Fusion Transformer, Random Forest Regression, and XGBoost Regressor
This study aimed to apply Temporal Fusion Transformer (TFT) to hourly global GPP upscaling by incorporating time-aware elements into the solution.
A TFT model of the ideal upscaling scenario, where past GPP measurements were presented for all areas, were implemented to benchmark the best possible TFT performance. For real-life upscaling application where not past GPP measurement were available, Random Forest Regressor (RFR), XGBoost (XGB) regressor models were used to establish baseline model performance, followed by two TFT modeling approaches:
-
Modeling with no past GPP measurement as feature input
-
Two-stage modeling with using estimated past GPP measurements from the predicted GPP values from either of the tree models.
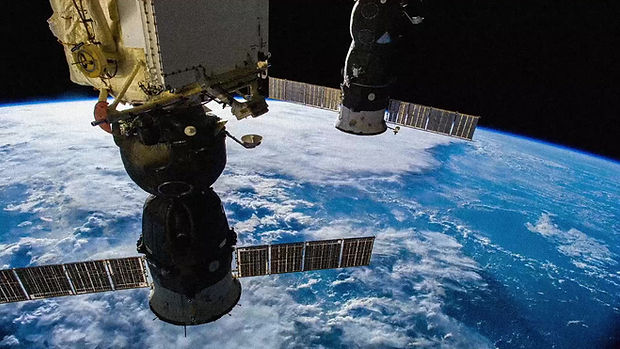
Result Analysis
In addition to examining model results and analyzing by IGBP using TFT's existing interoperability tools, a novel analytical approach was developed using TFT's explainability outputs. This method involved breaking down feature significance by encoder time steps to gain insights into the temporal influences of the features on model predictions.
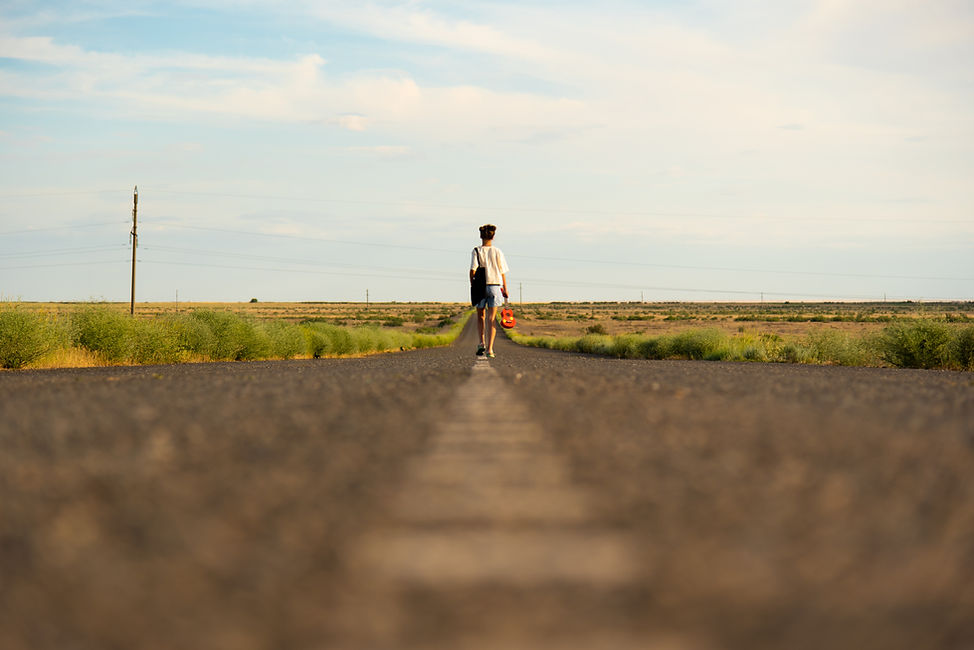
Look into Future
Multi-model approach by IGBP might be a promising solution to global GPP upscale.
Create global hourly GPP upscale data product based on improved model.